You’re getting this email as a subscriber to the BRXND Dispatch, a newsletter at the intersection of marketing and AI.
Luke here. Grab your Nikon tote bag and John Deere trucker hat because CES is in full swing. Here’s a giant autonomous tractor to whet your whistle.
What caught my eye this week (Noah)
I’m always careful about making too many predictions, as one of my core AI beliefs is that until we build up a lot more intuition for the technology, it will be impossible to fully comprehend the changes it will ultimately bring. With that said, I thought this was an interesting theory about AI going after “glue jobs”—the kinds of roles where the main objective is to translate something from one team into a different format for another.
In the past, I’ve talked a lot about AI as a fuzzy interface—basically meaning its single most important capability is to transform data from any format to any other format—but I hadn’t thought about the impact of that on the many jobs that exist to solve a very similar thing. There are a huge number of roles inside companies that exist to take information from one source, a customer, an engineering team, etc., and put it into a more digestible format for another (often execs). If this is a consistent enough process, I find it extremely believable that it could be replaced with AI fairly easily. I think this is part of why I think AI is coming for SaaS. Just like a lot of these jobs, much of the software ecosystem exists to reformat information.
What caught my eye this week (Luke)
CES was awash with agent hype this year. Jensen Huang called agentic workflows a “multi-trillion dollar opportunity;” Sam Altman said we might see virtual employees joining the workforce before the year is out.
But how do AI agents stack up on typical software work today? New benchmark testing shows that they can already autonomously handle about 24% of workplace tasks. Claude 3.5 Sonnet led the pack in performance by a lot, but it was also the most expensive model to run in terms of time and cost.
Microsoft plans to invest $80B in AI data centers in 2025. While this number might seem staggering, it actually represents a continuation of their current spending trajectory. The company invested $20B in the most recent quarter, which was a 79% increase year-over-year. This year, in a departure from recent trends, capex will actually be flat.
The cost of intelligence is coming down fast—faster than the cost of compute and storage fell for SaaS a decade ago. In March 2023, the cost of GPT-4 APIs was ~$30 per 1M tokens. Today, the cost of DeepSeek V-3 APIs, benchmarked at a similar level, is ~$1 per 1M tokens.
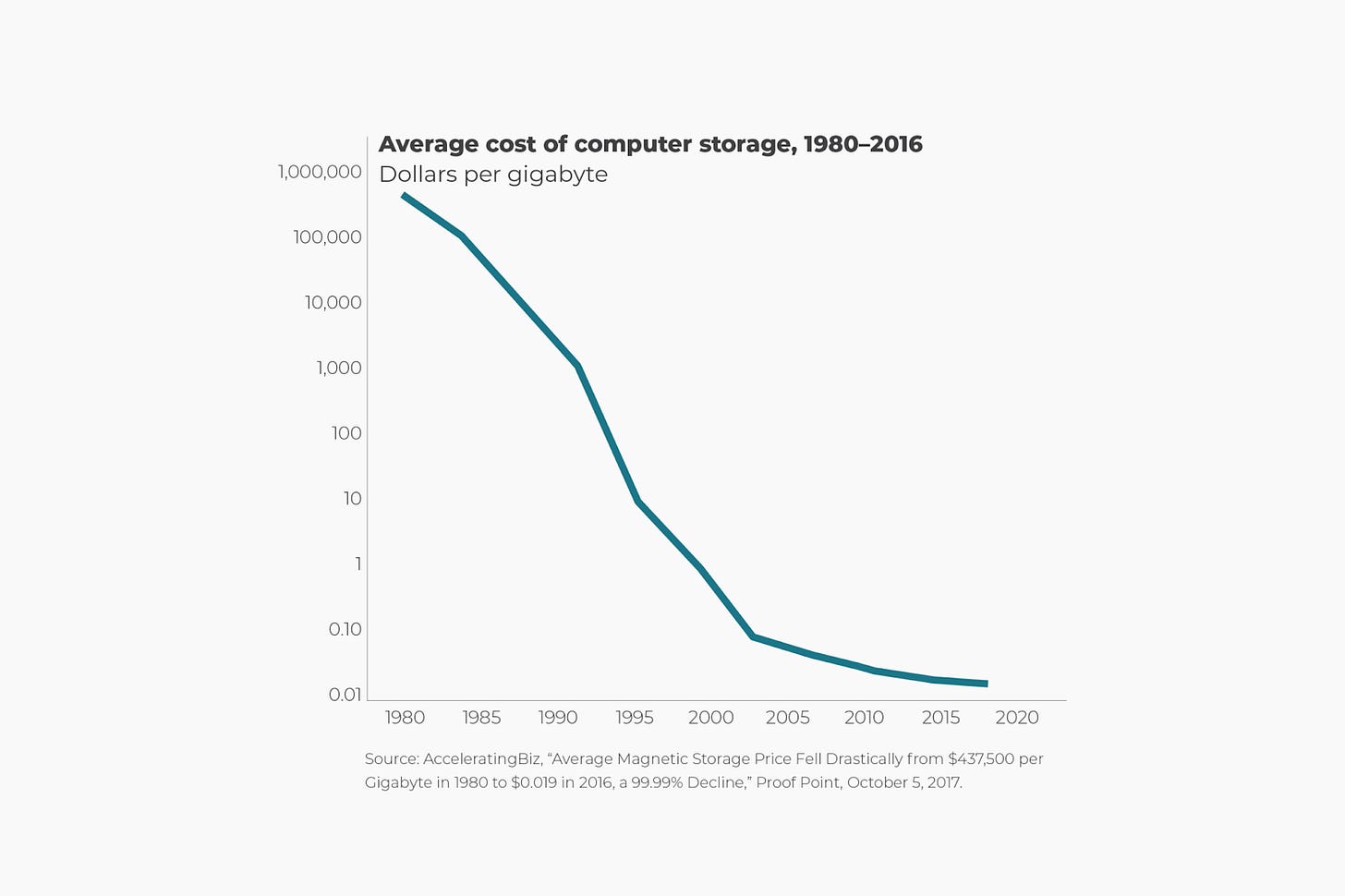
Search volume for the keyword “ai agency” is rising sharply. Everyone seems focused on building the next great AI SaaS tool, but the real value isn’t necessarily in the models themselves—it’s in the expertise required to implement them effectively.
Microsoft is open-sourcing its small but powerful Phi-4 model for developers to build on top of. It’s on Ollama if you want to test it on your computer.
Neuradocs is an AI knowledge base that lets you automate your Slack and Discord channels. This might be an interesting one for community managers to play around with.
I’ve seen a lot of people talking lately about MiniPerplx, an open-source alternative to Perplexity for searching the web, academic papers, YouTube videos, and tweets. Powered by Vercel and xAI’s Grok, it’s not as fast or accurate as Perplexity in my experience. But I do find it to be a good workaround for X’s flawed search functionality and other user experience issues.
As always, if you have questions or want to chat about any of this, please be in touch.
Thanks for reading,
Noah & Luke
I think about this a lot and I think your argument makes sense. I find that I spend a lot of time using AI to turn structured data into unstructured data or vice versa. Or sometimes it’s multi-step: ingest large amounts of unstructured data, identify trends in said data, structure and quantify. I think so much of what we do is 1. Sizing things (markets, probabilities, customer segments etc.) and then 2. Deciding courses of action, which are usually uniquely qualitative moments “Therefore we will do X”. I also think that as glue jobs go away we will have a period where much human work will be intense decision making (identifying and authorizing courses of action) before the Wall E world where the machines even make consequential decisions for us. Because the current corporate structures have mostly “glue workers” and relatively few decision makers, this may usher in a hyper-competitive environment where we have more companies, with fewer workers per company, engaged in intense competition around making better decisions. Production, in all its forms (industrial, digital etc.), becomes even more commoditized and only the decision about WHAT to offer remains as the principal domain of human competition. Regulated industries protecting themselves via “government oversight” will come under intense political pressure to drop barriers to entry (and indeed this is already happening with the new admin about to come on the scene in the US and soon in the UK, Canada etc.).