You’re getting this email as a subscriber to the BrXnd Dispatch, a (roughly) weekly email at the intersection of brands and AI. If you missed my conference in May, I have shared a few talks here, and the rest are up on YouTube.
With intrepid advertisers returning home from the south of France last week, AI talk again crescendoed. The cacophony of noise has somehow gotten even louder in the last twelve months. On the one hand, you have a crowd of folks, including Sam Altman, who are preparing the creative industry for their reckoning. Altman recently said he believes that AI will replace 95% of what marketers use agencies for in the coming years. On the other hand, there are creatives and technologists who are screaming from the rooftop that these things are incapable of true human creativity. As someone who has spent the last few years living at this intersection, I would say the truth is a bit more complicated. Or at least something is being lost in translation.
If you go into ChatGPT or Claude and mindlessly ask it to write a marketing headline or a poem, it will do a perfectly fine job. Not great, not terrible, just fine. By the very nature of the way we train these models, we should expect the output to be average. One way to conceive of these models' learning process is as a kind of flattening of knowledge: connections are strengthened and weakened based on their availability in the training corpus, leaving GPT-4 and the like with an incredibly down-the-middle tone. In some sense, they’re compression machines.
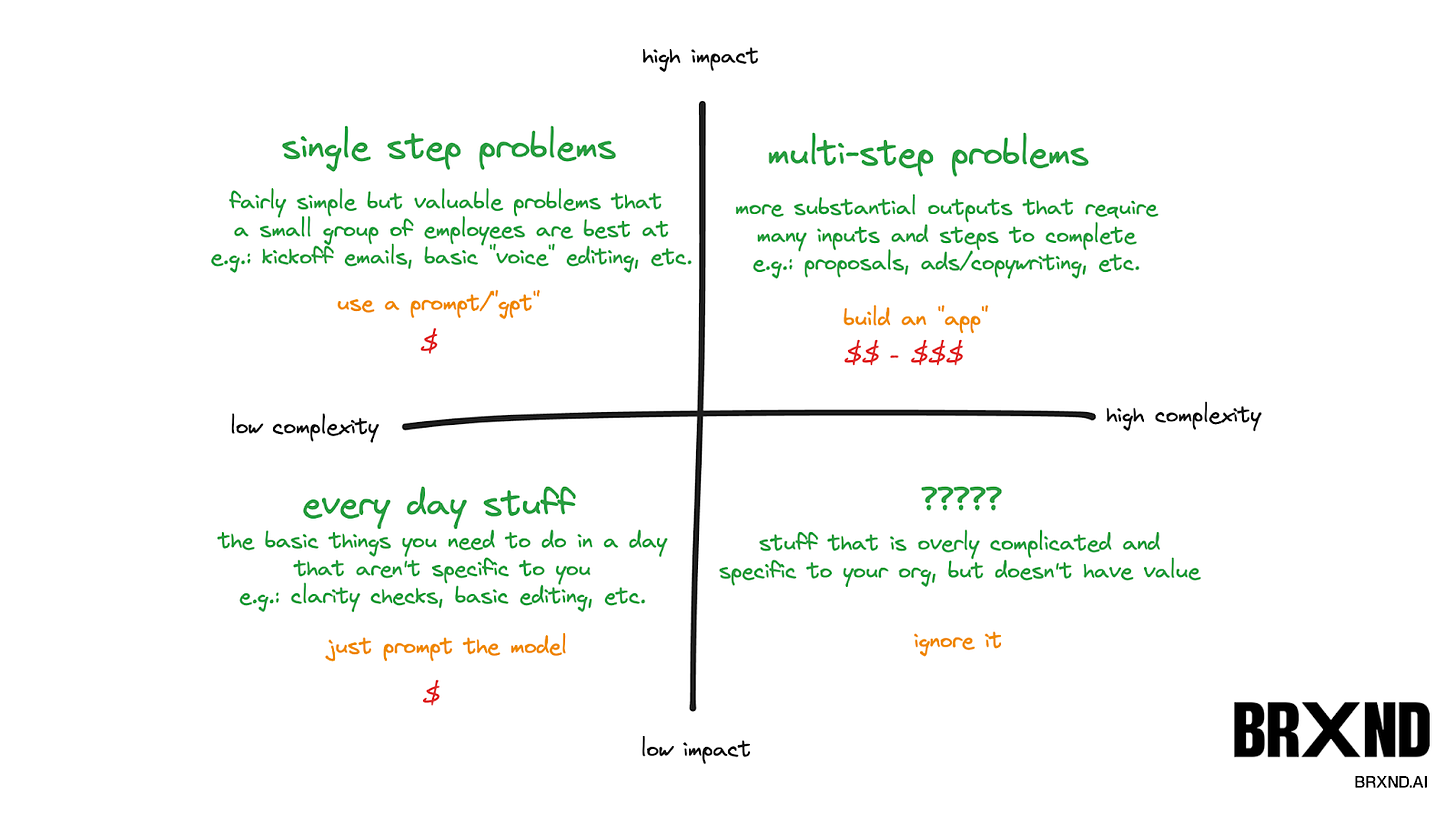
After the initial training, model makers fine-tune the AI through a process called Reinforcement Learning from Human Feedback (RLHF). Think of it like an editor refining a writer's work. Human evaluators review the AI's outputs and provide feedback, effectively teaching it to produce better responses. This process helps the AI align more closely with specific goals or quality standards. It's a crucial step in making the AI's outputs more useful and appropriate for real-world applications.
Unsurprisingly, brands don't pay advertising agencies millions to produce average work. So, the baseline output of these systems should, in theory, be reasonably far below what a WPP or Omnicom creative agency would knock out. But judging the output of these systems without spending time refining the input and steps it takes to produce quality output is like sitting a copywriter down with a brief and asking her for the final line after she finishes reading. Creativity is a process—often an incredibly confusing one.
What I've seen in my own work collaborating with creative experts of all ilk is that when you take someone who is both talented and self-aware of the process to produce great work, it isn't overly challenging to design a system that can produce very high-quality output. But sometimes, that system requires a winding path. In one project, I encountered an issue where the AI was being too literal in its interpretations, producing output that wasn't quite right for the intended audience. The solution wasn't straightforward—simply adjusting the prompt wasn't enough. Ultimately, I found that introducing a second AI to review and refine the first AI's output was the most effective approach. This “AI reviewer” acted as a filter, adding a layer of interpretation that helped align the final result more closely with the project's goals.
In another project, I designed a system that took a brief and produced creative output by utilizing a set of personas to give feedback throughout the process. This approach attempted to mimic the back-and-forth reality of creative work. Most recently, I worked on a tool that looked at the AI content I approved or edited and tried to find patterns in those changes so it could evolve the system's ever-growing style guide. This allowed the AI to learn from real-world creative decisions, gradually aligning its outputs more closely with professional standards. If you want expert-quality output, you’ve got to provide the system with expert-quality processes and inputs.
We shouldn’t be surprised it takes this kind of work to get exceptional output from these systems. The race to train and scale these models has left most of them starting from the same place: a massive scrape of the web called the Common Crawl. While this dataset is large and clearly capable of delivering a solid foundation, great creativity doesn’t come from consuming the same set of materials as everyone else. So now all these large AI companies are in a mad dash to find untapped wells of unique data—new ways to distinguish their model from their competitors.
But the biggest untapped source of data lives in the heads of the world's experts. While one path people fear has AI hooking us all up to Matrix-style brain straws, what’s actually happening is that those experts who are finding ways to combine their knowledge with the power of these models are getting enormous leverage.
Does this mean AI can produce highly creative output? Of course, it does. Is it easy? No. Will it ever be easy? I have no idea. Will this mean fewer jobs for creatives in the future? It could, for sure. Are all these reasonable questions? Absolutely. It seems clear to me that these models allow particularly talented people to amplify their output by 10 or 20 times.
Ultimately, AI's impact on creativity isn't a simple story of replacement or revolution. It's a nuanced collaboration between human expertise and machine capabilities. The most successful creatives will likely be those who learn to harness AI as a powerful tool in their process, amplifying their talents rather than replacing them.
As we navigate this new landscape, it's crucial to approach AI with both excitement and skepticism. The only thing I feel certain about is that you shouldn't trust anyone who too confidently predicts AI's future. This is a massively strange and powerful technology, and it will take years, likely decades, to fully understand its downstream implications.
For now, the best approach is to stay curious, experiment, and learn. This technology can be made to work at expert levels today (in text and with work, of course), and anyone claiming otherwise is not fully engaging with its current capabilities.
Thanks for reading, subscribing, and supporting. As always, if you have questions or want to chat, or if you’re at a brand and want to experiment with building some of this stuff, please be in touch. I am having a lot of fun working with folks on prototyping various creative AI tools.
Thanks,
Noah
Hey Noah. Enjoyed your article. As someone who helps companies think about the future, a lot resonated here.
What do you feel about the effect of all this compute on the planet. Humans will be creating orders of magnitude more content and data than anyone will consume. And the environment is set to suffer. I don't by into abundance and "technology will solve the problem over time". We're already seeing big tech companies backtrack on environmental commitments.
How do you think knowing we're destroying the planet should shape AI development and use?